New research using machine learning techniques shows that it’s possible to harvest minimal data from animal brains during periods of rest to shed light on how the brain forms and retains memories.
The researchers’ work employs hidden Markov models commonly used in machine learning to study sequential patterns. Their strategy analyzes waves of firing neurons that race in an instant across the hippocampus and beyond in animals while they’re active and, significantly, while they rest.
Mental maps
The firing patterns of neurons in the hippocampus—seahorse-shaped tissues in each hemisphere of the brain—have long been seen as important to the formation and storage of memories. Researchers detect and measure these patterns by placing electrodes into the brains to monitor them in real time.
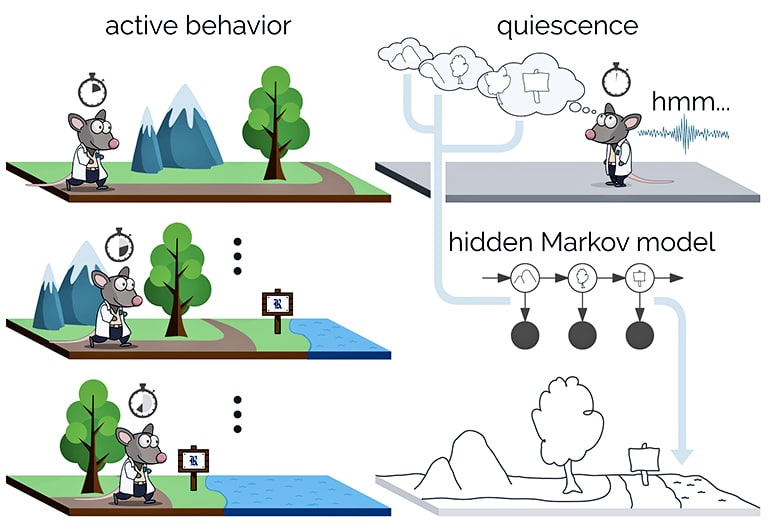
“Animals encode a memory of an environment as they run around,” says Caleb Kemere, an assistant professor of electrical and computer engineering at Rice University who specializes in neuroscience. “They form a spatial map as individual neurons are activated in different places. When they’re awake in our experiments, they’re probably doing that exploration process 40 to 60 percent of the time.
“But for the other 40 percent, they’re scratching themselves, or they’re eating, or they’re sort of snoozing,” he says. “They’re not asleep, but they’re paused; I like to call it introspecting.”
Those periods of introspection provided the critical data for the study that inverted the usual process of matching brain activity to movement while the animals were active. The researchers gathered the primary data over the course of many experiments under the direction of Kamran Diba, an associate professor and leader of the Neural Circuits and Memory Lab at Michigan Medicine.
As the animals explored either back-and-forth tracks or maze-like environments, electrodes in their brains sensed sharp wave-associated bursts of neural activity called population burst events (PBEs). In these events, between 50,000 and 100,000 neurons all fire within 100 milliseconds and send ripples throughout the brain that are not yet fully understood.
Experiments elsewhere had shown that PBEs included the activation of place cells in the hippocampus when an animal is in a particular location. These cells fire in a sequence that helps program the brain’s spatial and episodic memory, allowing the animal to build an internal map of its environment.
But the new experiments ignored all neural activity during active behavior or exploration and relied on data gathered only when the animals were paused—in total, about 2 percent of the time during experiments. The research team’s models were able to sort “recall” or “reactivation” bursts that appear to represent memories from other noisy signals in the hippocampus.
The researchers believe these signals in active animals presage the encoding of cells that make up place fields and show that in resting minds, PBEs offer a way to uncover a memory or spatial map without directly observing those cells in the locations that they would normally fire.
“We realized there’s enough data in those periods of reactivation that we can construct models from what the animals remember,” Kemere says. These turned out to correspond remarkably well with patterns represented by Bayesian analysis of theta waves generated while the animals were active, he says.
“When I was recording the data, I was mostly interested in neuronal activity during theta oscillations, when the animal was running,” Diba says. “However, the resting information turned out to be the most interesting aspect.”
Reconstructed memories
Markov models provided a template on which the pieces of a memory could be assembled. “Markovian dynamics simply state that you can predict your next step by only knowing your current step,” says Rice graduate student Etienne Ackermann, co-lead author of the paper. “You don’t need to know your entire past before that.
“In the brain, we think about these steps as underlying states that we cannot see directly,” he says. “They’re really hidden. But we can observe some proxy to those underlying states when we record electrical activity. It doesn’t tell us the internal state of the brain, but it can give us enough information to use a hidden Markov model to make a best guess about the sequence of the states.”
With enough sequences, the researchers were able to statistically recognize those that represent the memory of an environment, even when the experiments were unsupervised—that is, with no data that directly correlated brain activity with physical activity.
“I was surprised at how well it worked, and how much rich information about the environment was captured by the hidden Markov models,” says Diba, whose lab helped strategize ways to identify events.
“This is a really neat example of how we can use advanced machine learning techniques on brain data,” Kemere says. “It gives us the ability to see the structure of memory when those memories are being covertly expressed.
Evidence shows animals can play back memories
“With the hippocampus, you can normally see the structure of a memory by correlating it with animal behavior,” he says. “By using unsupervised learning, we were able to form that same structure from the periods when there was no behavior. This reveals an incredible richness in these covert memories.”
Kemere says the new models can be used to analyze existing sleep data sets. “We’ve known for a while that when animals sleep, reactivation and consolidation processes go on,” he says. “We can gather data but we really haven’t known what to do with it.”
He expects the models will help researchers sort forming memories from signals that represent dreams, noise, or even the essential process of forgetting useless data.
“Because we haven’t had a good way to quantitatively assess whether something is a good memory or a noisy memory, there are a lot of hypotheses about how sleep and reactivation should work that we haven’t been able to test,” Kemere says. “This is going to allow us to understand memory in places where we never have before.”
Protein calls up ‘reserves’ to make stronger memories
Researchers from the University of Michigan; the University of Wisconsin-Milwaukee; the University of Texas Southwestern at Dallas; and the University of California, Berkeley, contributed to this research.
The National Science Foundation, the National Institute of Mental Health, and the Human Frontiers Science Program supported the research. The team’s paper appears in the journal eLife.
Source: Rice University