To thwart deep fakes, researchers have developed an experimental technique to authenticate images from acquisition to delivery using artificial intelligence.
Determining whether a photo or video is authentic is becoming increasingly problematic. Sophisticated techniques for altering photos and videos have become so accessible that deep fakes—manipulated photos or videos that are remarkably convincing and often include celebrities or political figures—have become commonplace.
In tests, the prototype imaging pipeline increased the chances of detecting manipulation of images and video from approximately 45 percent to over 90 percent without sacrificing image quality.
Paweł Korus, a research assistant professor in the computer science and engineering department at the Tandon School at New York University, pioneered this approach. It replaces the typical photo development pipeline with a neural network—one form of AI—that introduces carefully crafted artifacts directly into the image at the moment of image acquisition. These artifacts, akin to “digital watermarks,” are extremely sensitive to manipulation.
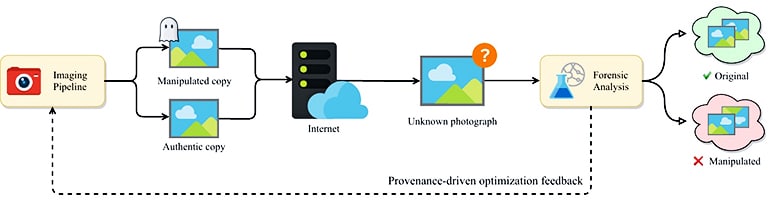
“Unlike previously used watermarking techniques, these AI-learned artifacts can reveal not only the existence of photo manipulations, but also their character,” Korus says.
The process is optimized for in-camera embedding and can survive image distortion that online photo sharing services apply.
The advantages of integrating such systems into cameras are clear.
“If the camera itself produces an image that is more sensitive to tampering, any adjustments will be detected with high probability,” says Nasir Memon, a professor of computer science and engineering and coauthor, with Korus, of a paper detailing the technique. “These watermarks can survive post-processing; however, they’re quite fragile when it comes to modification: If you alter the image, the watermark breaks,” Memon says.
Most other attempts to determine image authenticity examine only the end product—a notoriously difficult undertaking.
Korus and Memon, by contrast, reason that modern digital imaging already relies on machine learning. Every photo taken on a smartphone undergoes near-instantaneous processing to adjust for low light and to stabilize images, both of which take place courtesy of onboard AI.
In the coming years, AI-driven processes are likely to fully replace the traditional digital imaging pipelines. As this transition takes place, Memon says that “we have the opportunity to dramatically change the capabilities of next-generation devices when it comes to image integrity and authentication. Imaging pipelines that are optimized for forensics could help restore an element of trust in areas where the line between real and fake can be difficult to draw with confidence.”
Korus and Memon note that while their approach shows promise in testing, additional work is needed to refine the system. This solution is open-source and accessible here.
The researchers will present their paper at the Conference on Computer Vision and Pattern Recognition in Long Beach, California.
Source: New York University